June 2024, Vol. 251, No. 6
Features
Pipeline Integrity: Improving Data to Identify Selective Seam Weld Corrosion
Sponsored ContentBy Matt Romney, Senior Product Manager of Global Pipeline Integrity, T.D. Williamson
Nearly 40 years ago, an 8-inch gasoline pipeline failed north of Minneapolis, resulting in a serious incident with significant environmental and property damages.
In its investigation1, the National Transportation Safety Board (NTSB) said the pipeline, which had been hydrostatically pressure tested above its normal operating pressure two years earlier, had failed at a pressure below that.
Although the NTSB couldn’t say with complete certainty what caused the failure, investigators concluded susceptibility of the low frequency, electric resistance welded (LF-ERW) pipe to weld corrosion was a contributing factor. LF-ERW pipelines installed during a certain era often did not undergo a proper post-weld heat treatment, and NTSB believed the corrosion had weakened the weld.
To try to prevent something similar to this failure from occurring, the NTSB called for better data collection on pipeline leaks and failures, including those potentially related to selective seam weld corrosion (SSWC). SSWC occurs in the bond line of the longitudinal seam weld, where the corrosion attacks the long seam weld material preferentially compared to the surrounding base pipe material. The NTSB recommendations evolved into today’s pipeline integrity management requirements.
Though there have been a small number of SSWC-related events since 1986, fortunately, none have risen to the same impactful level. Much of the credit goes to coatings and cathodic protection that help keep corrosion at bay. In addition, improved pipe manufacturing practices have prevented LF-ERW pipeline material from being installed since the late 1960s and early 1970s.2 Even today, industry research organizations such as Pipeline Research Council International (PRCI) continue to research SSWC susceptibility and integrity management recommended practices.
Further, given that 80% of U.S. pipelines were already in place before 1970, the ability to detect, identify and size SSWC is just as important as ever — perhaps more so, since SSWC is a time-dependent threat with the potential failure risk increasing over time if the corrosion is still active.
In fact, the problem is so urgent that the Pipeline Hazardous Materials and Safety Administration (PHMSA) gives operators of hazardous liquid pipelines just 180 days to evaluate and remediate corrosion of or along the longitudinal seam weld after it’s been discovered, regardless of the threat posed.
Interacting Threats
SSWC can occur when a susceptible long seam weld is subjected to an active corrosion environment. Because of its susceptibility to preferential corrosion, the long seam weld material corrodes at a faster rate than the pipe body material — Kiefner and Associates said between two to four times faster3 — resulting in a deep V-shaped groove aligned with the long seam weld axis.
Due to its aggressive corrosion depth growth rate and orientation perpendicular to the primary stress, an SSWC anomaly poses a greater threat to pipeline integrity than a similar volume of general corrosion crossing the long seam weld does.
Kiefner also notes that SSWC is an interacting threat. Typically, interacting threats are defined as two or more coincident features or anomalies whose coincidence results in a greater integrity threat to the pipeline system than what each feature or anomaly poses individually.
In the case of SSWC, classic corrosion mechanisms occur coincident with a susceptible seam material. This interacting nature poses a greater threat risk for a number of reasons according to Kiefner, including:
- The axially oriented nature of SSWC may be difficult to find with conventional axial magnetic flux leakage (MFL) technology.
- When SSWC is detected, it may be difficult to characterize.4 In fact, SSWC anomalies in the long seam weld can sometimes demonstrate characteristics similar to trim defects or stable manufacturing anomalies, which are typically lesser integrity threats and often considered benign.
- To accurately classify the anomaly and deploy the appropriate resources requires the right in-line inspection (ILI) equipment and a very complex classification algorithm that has been refined by significant dig feedback.
One Not Enough
There’s no doubt that over the years, advances in ILI technologies have made it easier to detect and characterize anomalies in the long seam weld. Complex sensor arrangements on magnetizer assemblies that magnetize the pipe in complementary directions have been particularly useful.
There is a key reason why. SSWC features have a pair of nested feature geometries: In many cases, the seam weld, with its narrow, axially oriented slotting feature, is embedded in a field of general volumetric corrosion.
Further complicating matters, said T.D. Williamson (TDW) Principal Data Science Engineer Adrian Belanger, is the fact that the corrosion containing the selective seam weld corrosion can manifest itself in a number of ways.
For example, he said, it’s possible for a patch of corrosion to cross the seam without manifesting any preferential growth along the seam but contain deep, narrow, axially aligned grooves that follow the seam. Additionally, the corrosion could be preferential to the seam with only shallow SSWC notching within it. In both cases, using only a single direction of magnetization can mischaracterize the corrosion or miss it altogether.
“Because the conditions vary so widely, it’s extremely difficult, if not impossible, to consistently distinguish between SSWC and general corrosion crossing the long seam by using a single magnetization direction,” Belanger said. In essence, regardless of the number of sensors thrown at the problem, there is only so much information to be gleaned from a single magnetization direction.
“It’s not what you see, it’s what you don’t that matters,” Belanger said.
Improving accuracy requires multiple magnetic field orientations, and one of those fields must be sensitive to long, axially oriented, narrow pipeline defects. Using multiple magnetic field directions provides additional context about the actual anomaly geometry and position.
Spiral MFL (SMFL) is the only technology that generates a helically oriented (spiral or corkscrew-shaped) field in the pipe wall. Beyond the general detection of the long seam, the SMFL data provides a complementary view of the SSWC defect — “kind of like taking a photo from two different perspectives to create a 3D image,” Belanger said.
Figure 1 displays the axial MFL and SMFL data streams for a section of pipe, the long seam weld is typically very clear in the SMFL data stream but much harder to detect in the MFL data.
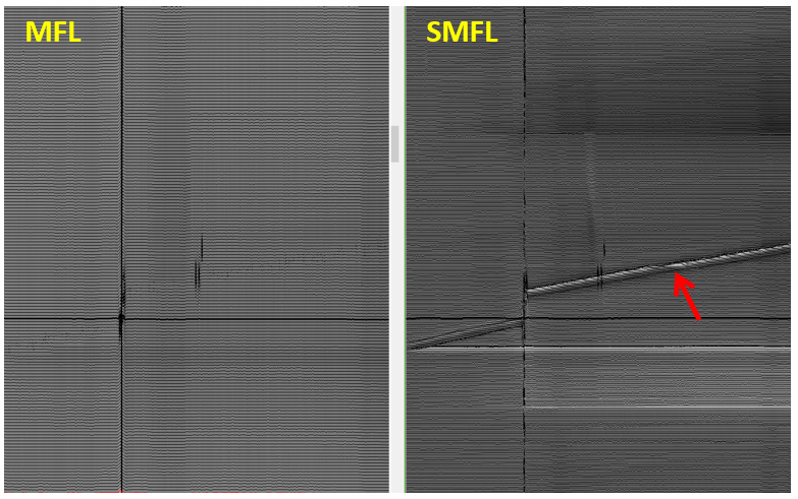
As another example, Figure 2 shows the circumferential profile of an SSWC feature for MFL and SMFL datasets. The ILI tool sensor data is on the x-axis, and the median centered gauss values recorded by the tool’s Hall effect sensors are on the y-axis. (Hall sensors are sensitive to the strength of the magnetic field. As the magnetic field increases in intensity, the voltage returned from the Hall sensor changes.)

The MFL profile is indicative of a general volumetric corrosion feature with a smooth, parabolic-like shape. Conversely, the SMFL has a distinct peak within the area of general corrosion indicating that a narrow axial feature is also present.
Without the SMFL dataset, there would be no indication that a narrow axial feature is present.
TDW Data Science Engineering Manager Robert Coleman said that SpirALL MFL, one of six primary sensor technologies on the company’s MDS Pro platform, “provides a more thorough look at axial features, particularly in or near the seam weld. It enables a more robust assessment of the signal than traditionally axial fields.”
The MDS Pro platform simultaneously delivers multiple data streams to provide a comprehensive analysis of the pipeline. In addition to SpirALL MFL, the MDS Pro platform includes:
- Axial MFL, which is sensitive to geometric general metal loss features such as pits, general metal loss and circumferential slotting and gouging. Given that traditional MFL field direction is parallel to the SSWC anomaly, the field response has a lower sensitivity to axially oriented anomalies, but can identify the corrosion on the long seam that SSWC can initiate in.
- IDOD sensors are sensitive to the location (internal surface/external surface) of general metal loss anomalies.
- Low-field MFL (LFM) produces a low-strength magnetic field in the pipe wall and, as such, is sensitive to changes in material permeability. Among other things, LFM technology can be used to assess permeability changes due to the pipe manufacturing process. LFM signal changes coincident with an SSWC anomaly could indicate an additional feature or atypical condition.
- High-resolution geometry characterizes dents, expansion, wrinkles, ripples and general changes to the pipeline’s internal diameter (ID) bore. Geometry data can be used to assess the presence of trim or long seam weld anomalies that could contribute to MFL/SMFL data signals of the pipeline. Geometry data can be useful for characterizing certain seam types such as electric flash weld (EFW) pipe, which is known to be susceptible to SSWC.
- High-resolution mapping documents the center line of the pipeline. In recent years, this technology has grown in popularity for bending strain and line movement analyses.
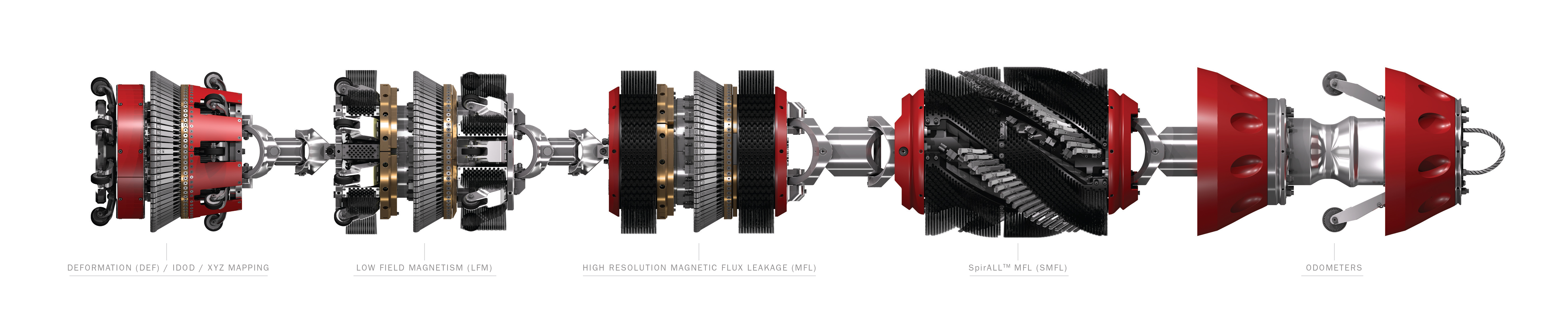
First SSWC Classifier
Considering that MFL field is less impacted by axially oriented anomalies, comparing its data to that of SMFL improves the characterization of SSWC anomalies.
In fact, the difference in signal response between MFL and SMFL was critical to the Department of Transportation (DOT)5 project that eventually led to the development of the industry’s first validated SSWC classifier by TDW.
The classifier leverages the data collected by the MDS Pro platform to characterize long seam weld corrosion anomalies. It utilizes an algorithm based on the amplitude and width of both MFL and SMFL signals.
The project noted a large SMFL amplitude response that was sharply narrow in the width direction as an indicator that the anomaly was circumferentially narrow and axially oriented. When the SMFL signal response is uncharacteristically large compared to the corresponding MFL response at the same location, the anomaly is more likely to be SSWC.
To validate the model, TDW analyzed about 700 SSWC calls made on 75 ILI runs between 2013 and 2021. Dig feedback data was available for 201 of these calls, and NDE only found three SSWC anomalies that were not reported as SSWC by the classifier.
Additional features often misidentified by ILI were used as controls during validation, including general corrosion crossing the long seam weld, lack of fusion (LOF), mill anomalies, long seam weld variations, axial planars and linear indications. The validation process demonstrated the classifiers’ ability to accurately classify features as SSWC, distinguishing them from features that might create similar signatures in MFL data alone.
Since TDW introduced the classifier, it has been used by operators across the globe to properly classify seam weld corrosion anomalies as either SSWC or general corrosion coincident with the long seam. The validated performance specification allows operators to confidently characterize the integrity risk of each anomaly and prioritize their mitigative actions.
Seeing the True Nature of SSWC
Key to the success of the TDW classifier is the approach leveraging two separate high-field MFL technologies, axial MFL and SMFL, to characterize the seam weld corrosion anomalies.
The axial nature of the SSWC feature limits the field response that is achievable with a pure axial MFL tool configuration, independent of the axial tool sensor resolution. Improved classification is achieved with a magnetic field crossing the axial SSWC anomaly, characterizing the true axial nature of SSWC anomalies when present.
With better data, pipeline operators can figure out what they’ve been missing. The result is safer, more compliant systems.
[1] https://www.ntsb.gov/investigations/AccidentReports/Reports/PAR8702.pdf
[2] https://www.phmsa.dot.gov/data-and-statistics/pipeline-replacement/decade-inventory
[3] https://kiefner.com/selective-seam-weld-corrosion-how-big-is-the-problem/
[4] https://kiefner.com/selective-seam-weld-corrosion-how-big-is-the-problem/
[5] https://primis.phmsa.dot.gov/matrix/PrjHome.rdm?prj=498
Comments