October 2017, Vol. 244, No. 10
Features
Teaching Technology: Solution Finds Small Pipeline Leaks
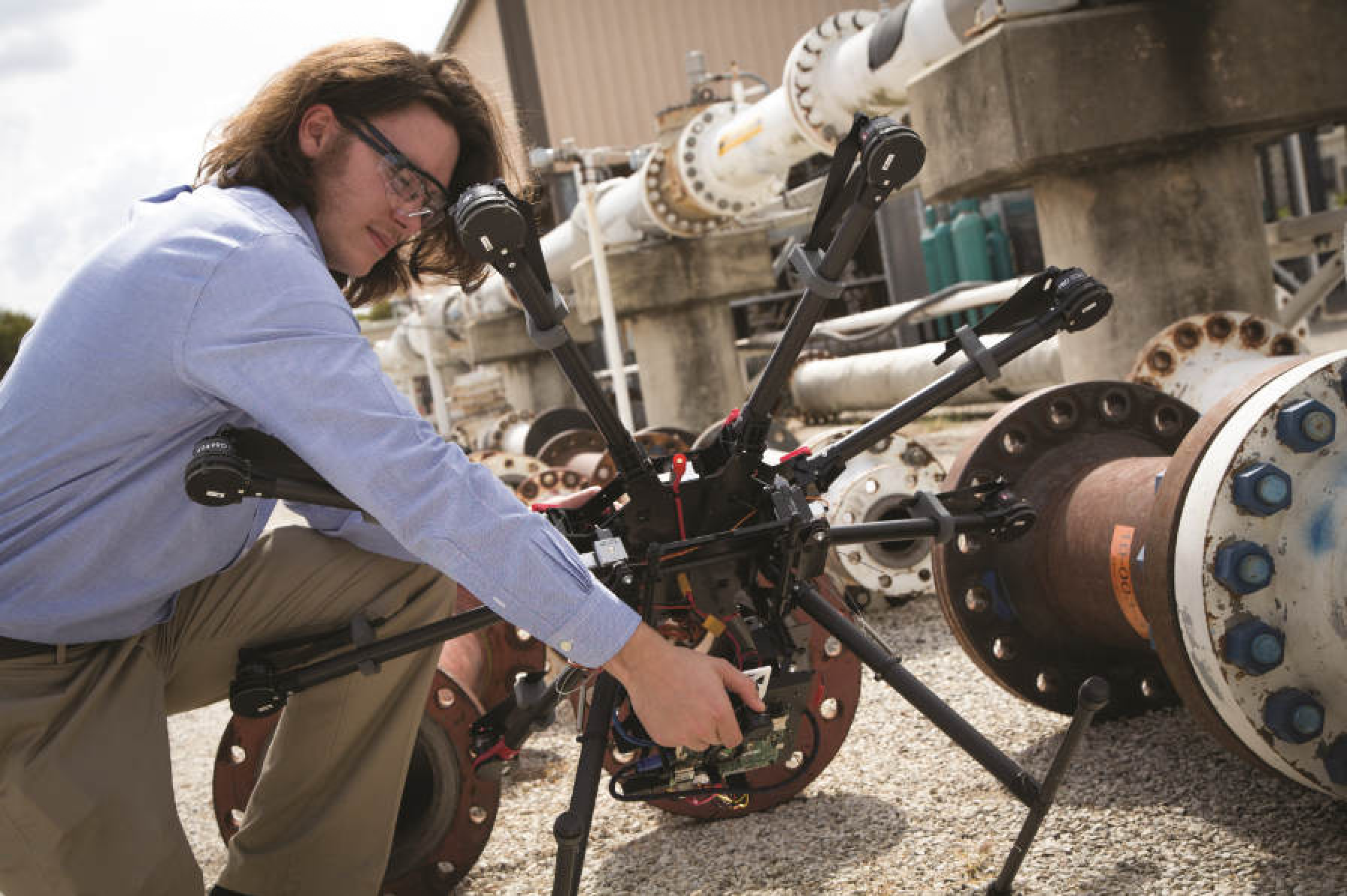
The domestic boom in oil and gas production has helped the U.S. increase energy independence in the past decade, but the benefits have come with environmental challenges. Among those are increased risks of hazardous chemical spills and methane gas leaks, which contribute to climate change by trapping heat in the atmosphere.
Between 2007 and 2012, leaks in the U.S. liquid pipelines network exceeded 100,000 barrels a year. That’s a pool of petroleum the size of the Lincoln Monument’s reflecting pool every two years. This volume is a 3.5% increase from the previous five-year period.
Southwest Research Institute (SwRI) in San Antonio is attacking the issue, developing technology that can autonomously pinpoint small liquid and gas leaks before they become major problems.
“It makes both environmental and economic sense to identify the smallest oil and gas leaks as early as possible to help ensure environmental safety and pipeline system reliability,” said Maria Araujo, a manager in SwRI’s Intelligent Systems Division. “With sensor fusion and machine learning techniques, we are using a branch of artificial intelligence to trigger rapid responses.”
Small Leak Detection
Working with SwRI’s Mechanical Engineering Division, Araujo led an internal research project that addresses gaps in technology for detecting small leaks in liquid pipelines. The Smart Leak Detection (SLED) system uses algorithms to process images from sensors scanning the infrastructure. These sensors can be positioned at sensitive pipeline junctures or deployed on drones to cost-effectively fly over pipeline networks.
Current practices in hazardous liquid leak detection include visual monitoring and external and internal leak-detection systems (LDS). Visual monitoring can entail pilots surveying infrastructure from a small plane. This technique is somewhat effective, but expensive and time-consuming, and cannot distinguish a puddle of water from a hydrocarbon spill. Existing external leak-detection systems do offer some benefits, including the ability to locate a leak, continuous operation, significantly better sensitivity than computational methods, and the ability to estimate the size of a leak, among others.
However, several drawbacks in existing LDS include high costs and difficulty in retrofitting existing pipelines to support these technologies. Some of these technologies are not effective for both under- and aboveground pipelines or when a very large number of sensors are required. And both visual techniques are less likely to find a leak the smaller it is.
Today, the predominant internal leak-detection systems used by the oil and gas industry rely on computational pipeline monitoring, or CPM, which looks for anomalies in flow volume and pressure. This process works well when identifying large leaks, but often fails to detect leaks of less than 1% of the pipeline flow volume. To put that into perspective, 1% of the Keystone XL Pipeline volume is about 8,000 bpd; today’s standard technology is neither accurate nor effective at pinpointing such leaks.
The crux of the problem is that CPM leak-detection systems tend to trigger false alarms with leaks of less than 1%. Historically, high false alarm rates have led system operators to ignore those alarms. Consequently, that means energy companies often don’t find out about leaks until property owners, or the media, report significant spill incidents. Nearly half of all leaks today are found by accident, not by leak-detection technology.
“It’s too late at that point; the damage is already done, so we need more reliable leak detection,” Araujo said. “With more than 2.5 million miles of existing pipelines, this is a major challenge for our energy infrastructure.”
Leak Signatures
The SwRI solution is multiplatform; it can be deployed on stationary platforms at pumping stations, located about every 50 miles along the pipelines. These are high-risk areas for leaks because valves and other specialized equipment can fail. But the technology can also be deployed on drones, to fly over long stretches of pipelines between stations. The SwRI solution fuses inputs from low-cost optical sensors and applies machine-learning techniques to reliably detect the chemical “fingerprints” of small hazardous liquid leaks.
The technique for fingerprinting chemicals uses the reflectance response of typical hydrocarbons using infrared and visual sensors. Cameras have been used to detect leaks before, but the SwRI team used artificial intelligence techniques to vastly improve the accuracy.
The team then used feature extraction and classifier training techniques to teach computers to identify unique features across the full range of environmental conditions. It not only provides a more reliable fingerprint of small liquid leaks, but also characterizes several non-leak events over the full range of operating conditions. This substantially reduces false positive rates.
Learning Techniques
Accuracy is paramount. Consider if a system identifies a pipeline leak in rural Alaska. To send a team of technicians out in the field to repair the leak is costly, even more so if they arrive and determine that it was a false alarm. Artificial intelligence is key to the accuracy of SLED.
Using machine learning, a computer can learn without explicit programming, by consuming copious amounts of data. The team collected thousands of images of mineral oil, crude oil, gasoline, diesel, and water on various surfaces, including gravel, grass, and concrete. These images were shot at various angles and under all conditions, from bright sunshine to cloudy skies, under a full range of temperatures. All of that data were fed into an algorithm to identify patterns that differentiate one liquid from another.
In addition to standard conventional machine learning approaches, the team applied advanced deep-learning neural network techniques. Deep-learning refers to the depth of layers that make up a neural network. The depth of these networks has traditionally made training and classification impractical.
However, recent mathematical discoveries combined with rapid improvements in multi-core processing hardware, have brought the potential of deep-learning techniques into the mainstream. In computer vision, one primary deep-learning technique is called convolutional neural network (CNN), a computational approach inspired by the organization of the animal visual cortex.
This flexible technique dynamically addresses the standard battery of computer vision challenges such as detection, classification, and tracking. SwRI developed and applied a deep convolutional neural network to process the data and identify hazardous liquids. Initially, the plan was to differentiate hazardous liquids from a puddle of water, but the system proved powerful enough to distinguish between the various petroleum products, identifying a gasoline leak versus a pool of diesel.
The result is a fully autonomous system that can be used with no human intervention, Araujo said. The system identifies leaks of crude oil, diesel, gasoline, and mineral oil under different lighting and weather conditions, accurately characterizing the signatures of leak and non-leak events.
Technology Transfer
The success of the internal research project helped SwRI land a federal Department of Energy award to apply the same technology to detect small gaseous leaks, specifically methane.
Methane, a primary component of natural gas, is considered a greenhouse gas because it absorbs the sun’s heat and warms the atmosphere. The SwRI team will develop an autonomous, real-time methane leak-detection system for the National Energy Technology Laboratory (NETL).
“Historically, it has been challenging to effectively detect and mitigate small methane leaks in real time unless you have inspection personnel with sensor devices stationed 24/7 across energy infrastructure,” Araujo said.
The Smart LEak Detection/Methane technology, also known as SLED/M, will be designed to automate small leak detection across the entire natural gas supply chain from extraction and storage to transportation and distribution. The two-phase DOE project will take place over 18 months.
In Phase I, SwRI will adapt its hazardous liquid spill detection capabilities to develop the methane detection system. Using integrated optical sensors and a GPU-based embedded processing unit, the new technology will create algorithms that learn to recognize patterns and trigger alarms during leak events.
Beyond the DOE project, Araujo’s team is developing new applications for the technology, including remote satellite sensing to identify oil spills in places like the Gulf of Mexico.
This article, reprinted with permission from Southwest Research Institute, originally appeared in the Spring 2017 issue of Technology Today, SwRI’s quarterly magazine.
Comments