February 2021, Vol. 248, No. 2
Features
Data Analytics, Predictive Maintenance of Field Automation
By Whitney Vandiver, Compliance Specialist, NuGen Automation
The modern world of pipeline operations was precipitated by the arrival of SCADA systems and field automation in the 1960s. The last six decades have experienced an explosion of technological development, with the most recent focus being on data analytics, and the pipeline industry has more than dipped its toe in the benefits of data analysis.
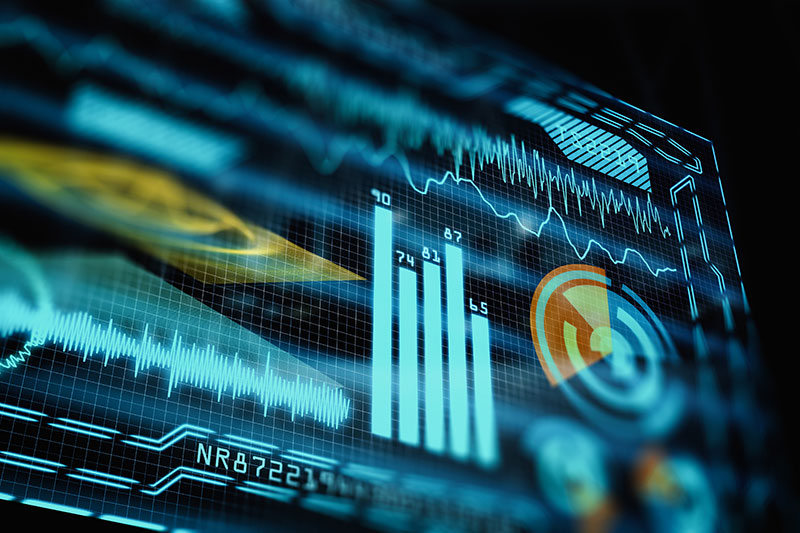
However, many operators have yet to understand how data analytics of SCADA data can not only help them understand their operations but influence their maintenance processes and reduce their overhead through predictive maintenance.
Pipeline operators frequently use a calendar-based maintenance approach, involving regular check-ups and rarely considering data outside of occasional system reports.
While operators can effectively plan maintenance activities via this strategy, many of them end up with overstuffed maintenance schedules with high price tags. When considering the long-term performance of assets, such calendar-based maintenance is in some cases unnecessary, having little to no direct impact on the assets’ continued operation in as much as 70% of cases.i
Unfortunately, maintenance that is performed after equipment downtime has begun not only increases operational costs but often leads to consideration of data that were available but overlooked before the failure occurred.ii
This post-failure strategy of unplanned maintenance on complex systems continues to plague the integrity of pipeline operations,iii and it is the gap between automation operations data and maintenance data and its direct impact on the effectiveness of maintenance efforts that has led the industry toward a more efficient strategy for pipeline maintenance for field automation and integrity management.iv
Analytical Maintenance
Pipeline maintenance strategies have evolved throughout various industries along with advances in technology. Reactive maintenance, which occurs when an operator reacts after a failure has occurred, is considered the classic strategy and involves no premeditated tactic.
This method advanced into preventative maintenance, which occurs when operators maintain equipment and systems at prescheduled intervals in an attempt to get ahead of potential failures.v
As systems advanced, operators made efforts to understand equipment’s performances and introduced condition-based maintenance, which looks for conditions that could indicate changes, damages or misconfigurations to evaluate current equipment status and allow for early maintenance.vi
Despite its understanding of how certain conditions can inform maintenance schedules, condition-based maintenance does not include the insight into operations that can be captured by data analysis.vii
Predictive maintenance builds on the previous strategies and goes a step further by integrating process data and applying analysis to anticipate equipment performance and identify how degradation will lead to failures – with the goal of performing maintenance before any failure-indicating conditions occur.viii
In other words, this strategy will inform an operator of how a device is likely to perform and how maintenance can be used to achieve maximum uptime and prolong operational life without the requirement of first encountering conditions indicating a move toward failure.
By using a comprehensive set of operational data, predictive maintenance captures the health of equipment to allow operators a more realistic look at their operations to see how devices are functioning in the moment rather than after warning conditions begin to occur.
Predictive maintenance comes with multiple benefits that have a direct impact on operations as a result of the strategy’s methodology, which includes failure prediction and diagnosis, classification of failure types and maintenance recommendations.ix Operators can better schedule maintenance in light of analytical data, leading to more applicable maintenance procedures without a swollen maintenance schedule.
This reduces unnecessary maintenance on equipment that has been traditionally maintained according to an industry- or manufacturer-recommended schedule as opposed to unique operational data.
By simplifying the maintenance schedule and focusing efforts on equipment with timeliest needs, maintenance overhead is also positively affected by eliminating superfluous upkeep.x
Moreover, downtime is minimized thanks to improved efficiency and the ability for technicians to anticipate issues specific to equipment before they begin to appear.xi While each operation is unique, predictive maintenance strategies have been estimated to lower maintenance overhead by 25%, decrease downtime by 70% and improve productivity by 25% on average.xii
Maintenance Cycle
Pipelines are complex systems in which devices are often dependent on each other, translating into a cascading failure if the conditions are right. Such complex systems can be difficult to manage when it comes to assessing relevant maintenance in advance of abnormal operations.
This issue has been partially addressed with SCADA technology; however, a controller can only take in so much input, and a good deal of the analysis needed to anticipate a potential equipment issue is often beyond the human brain’s capability. To do so, operators require a higher order of analysis that is embedded in a decision-making framework like that provided by data analytics.xiii
The term data analytics encompasses a wide variety of applications. Even within the oil and gas industry, data analytics can provide insight into several operational arenas. Its most powerful application, however, has proven to be with SCADA, which provides a plethora of data relating to equipment status, response and influences that lead to device behavior.
It is through machine learning that such analytics can translate this data into actionable items for operators seeking a predictive maintenance strategy for their assets. Machine learning utilizes algorithms to analyze historical data, capture patterns that relate to behavioral outcomes and forecast potential scenarios.
In the case of SCADA data, an algorithm assesses, in some cases, millions of data points and identifies patterns in how equipment responds to input, the resulting output and the relationships between these and other operational factors, such as component degradation, device failure and necessary maintenance intervals.xiv
In pipeline applications, four analytical categories are applied. Descriptive analytics considers what is occurring by understanding the context in which the data are supplied. This is already achieved in most operational control centers via HMIs, where controllers are provided with visualizations of operations.
Diagnostic analytics assesses this information via statistical analysis to provide insight into why an event occurred, which is most useful for identifying anomalies. This feeds into predictive analytics, which uses mathematical models to infer what is likely to happen in the future amid constantly variable conditions.
This tier can be furthered by prescriptive analytics, which creates models for how operators should respond to a forecasted event – in other words, recommending specific maintenance.xv
The first three categories of data analytics are key to providing predictive maintenance applications in pipeline operations, and each tier replaces a stage of assessment in the maintenance management cycle often used by operators today.
Descriptive analytics replaces the situational evaluation that occurs during reactive maintenance, looking at what failed and the situation surrounding the failure.
As we’ve already discussed, this is the simplest form of maintenance, which is why it is also the foundational tier of analytics. Diagnostic analytics replaces the root cause analysis where a larger understanding of the situation is ascertained.
Growing Stale
It is often at this stage that traditional maintenance strategies begin to grow stale as operators grow content understanding what precipitated a failure and attempting to address that information by applying a maintenance interval or procedure for a condition-based strategy.
Predictive analytics, however, takes operators beyond that stage and looks at how an asset has behaved in the past and how that behavior is likely to continue and evolve – and how that will likely affect equipment performance.
Data analytics for maintenance management not only replaces the benefits of the traditional, non-technology-based methods but enhances operators’ abilities to predict where maintenance is likely to be needed and when.
Moreover, because machine learning is a continuous process, such an application will continue to improve over the life of an asset by analyzing new data to learn an asset’s behavioral patterns.
The potential for the layer of prescriptive analytics is well-founded in pipeline operations in many areas. As technology develops and algorithms mature, operators might see the integration of such information into operational control center HMIs, whereby detected patterns and identified anomalies can be used to warn controllers of potential issues in time for operations to be adjusted to avoid the more common abnormal operations scenarios, such as automated emergency shutdowns of assets.xvi
This level of analytics would move the oil and gas industry beyond the generation of predictive maintenance into the world of prescriptive maintenance.
Data Analytics
While the most obvious application of predictive maintenance via data analytics is with field automation, a clear application that is often overlooked by operators is with integrity management.
Just as the application of data analytics has the potential to provide advanced warning to controllers to avoid potential scenarios, the reach of such predictive maintenance applications also carries the probability of performing a similar function for pipeline integrity management.
In the same way that predictive maintenance is able to identify trends and forecast scenarios relating to devices, it can also assist in identifying trends in pipeline integrity via the same devices it is helping to maintain.
Pipeline integrity management presents a complex data set when it comes to risk assessment. Traditionally, integrity schedules are based on intermittent data and industry standards in lieu of real-time pipeline data.
Often, operators must rely on data acquired from inline inspections and smart pigging systems, and some operators can gleam additional information from advanced leak detection systems. However, this information is often collected at prescheduled intervals, barring incidents that indicate the need for or require pipeline assessments.
While this information is useful and has proven to be foundational to pipeline integrity management programs, the unused time between the intervals can be costly if operators are unable to assess an asset’s integrity before it reaches a stage of degradation.
Moreover, traditionally used data sets that point to the same information can come from various systems or sources, which requires operators to align the data for a comprehensive picture.xvii
Collectively reviewing this information and connecting the dots can be a time-consuming process that is often slowed by heavy integrity schedules, leaving operators at risk of missing key indicators of integrity issues.
Data analytics, however, provides a solution to not only combining disparate data sets but pulling the most up-to-date field data into integrity risk assessments.
In the same way that data analytics applications can help us field data to predict issues with devices, they can also analyze relevant data to understand how pipeline integrity is reflected in certain pipeline behaviors.
Pipeline data, such as SCADA data for metering and instrumentation, can be continuously fed into a data analytics application, providing minute-by-minute information to inform predictive models.xviii
Patterns that potentially indicate the development of conditions that could produce leaks or ruptures – which are captured in some advanced leak detection systems – are the most obvious applications.
However, operators will benefit from more sophisticated predictions, such as patterns that indicate corrosion or weakening pipe, which is often only captured in scheduled inspections or realized after an incident has occurred.
This level of predictive maintenance offers a new generation of integrity management by allowing operators to see how their assets are aging as a process rather than as time stamps.
Because of integrity management programs’ objectives to monitor asset conditions, which are constantly being influenced, data analytics will not replace traditional integrity management practices. Rather, it will enhance operators’ abilities to understand their assets and to reduce risk via a more informed maintenance program.
Author: Whitney Vandiver is a compliance specialist with NuGen Automation, where she specializes in control room management compliance and assists operators with state and federal CRM audits. Previously, she worked for a pipeline operating company in compliance-driven documentation and served as a volunteer editor for the drafting of API MPMS 18.2. She holds a bachelor’s degree from the University of Central Oklahoma and a master’s and doctorate degree, both in linguistics, from Purdue University.
Comments