August 2021, Vol. 248, No. 8
Features
Live Mapping of HAZ Softening in Pipeline Seam, Girth Welds
By Mahyar Asadi, Advanced Welding Engineer, Applus Engineering Canada
Modern pipeline grades, such as X70 and X80, improve many properties but come with undesirable features such as heat-affected zone (HAZ) softening in seam and girth welds.
These pipeline steels with microalloying elements are manufactured using thermomechanical controlled processing (TMCP) with controlled rolling and cooling for improving mechanical properties. When pipelines are welded together, the HAZ material adjacent to the weld pool experiences an irregular thermal profile resulting in adverse microstructural evolution.
This relatively small zone undergoes a significant transformation starting with the transformation of ferrite to austenite, followed by grain growth and precipitate dissolution, and finally, austenite decomposition to different transformation products as the HAZ starts to cool down.
The change in the microstructure of HAZ is very diverse as one moves away from the fusion line, due to the different peak temperature and cooling rate experienced. Most pipeline welding production has more than one pass; for example, in the case of dual-pass welding, the interaction between HAZ of multiple passes results in a graded microstructure.
This graded microstructure builds a zone with various properties ranging from softer to harder than the base metal, with variation in both toughness and yield strength. Some regions in the HAZ can soften, resulting in strain localization and act as a potential region of failure. The extent of softening depends on the chemistry as well as welding parameters.
While welding parameters can control and minimize the problem in various design and production stages, the absence of assessment tools for HAZ softening limits the abillity of engineers to explore multiple welding scenarios using available time and reasonable cost to set up and control optimal welding.
Heat-Affected Zone
Industry 4.0 brings a leap forward from traditional automation to fully connected and intelligent manufacturing systems (Deloitte, 2017) by incorporating cyber-manufacturing.
The brain of the cyber-manufacturing platforms consists of digital twin (DT) systems that are the digital representation of production. This DT is designed to act as the system’s brain to make wise decisions through data communication with the digital muscles embedded in the production line.
Constructing a DT can take two general forms, data-driven DT and knowledge-driven DT. The data-driven DT relies on extensive data availability. Therefore, it is suitable for applications with mass productions such as automotive, electronics and textiles to generate large data sets at a low cost.
In contrast, the knowledge-driven DT uses the physical understanding of the processes to reduce the data required for prediction. The pipeline production does not have a large data set on HAZ on which to draw, and the cost of generating such an extensive data set is high for HAZ. This data deficiency limits the application of AI and digital twins for controlling HAZ softening in pipeline production.
Heart of Digital Twin
Using digitalization tools for constructing a DT of the production is limited by computational time for live production applications. Using machine learning (ML) advantages, simulation tools and ML have been integrated to solve this problem, where ML algorithms emulate the behavior of the time-consuming simulation for live analysis of HAZ during the production.
The integration of ML and simulation provides a platform for theory-training ML (also known as physics-guided ML), where one can construct a knowledge-driven DT using limited data rather than relying on a large data set.
We built a quick learner using a physics-guided ML approach in conjunction with an active learning strategy to enable our DT to conduct live mapping of the HAZ properties during multiple welding. Our quick learner is now mature in predicting HAZ properties for given arbitrary welding parameters in the production envelope of the pipeline.
Active learning is the backbone of our methodology that empowers us to reach the maturity level with fewer than hundreds of data labeling using physical and computational experimentation for a given material.
Live Mapping
We constructed a DT of X60 pipeline production with two submerged arc welding (SAW) weld passes (root and cap) with quick mapping capability of HAZ softening. This continuous map presents the spectrum of change in yield strength, ultimate strength and hardness. Additionally, we are now working with a pipeline production customer to include toughness property to the design envelope of HAZ control.
In contrast with many algorithms that need a large data set to make a prediction, our approach uses a wisely selected small set of 48 data points to characterize extensive welding scenarios through peak temperatures and cooling rates.
These data points are the representative thermal profiles consisting of two peak temperatures from root and cap welding with the nonlinear characteristic cooling rates for the pipeline production. The selected set of thermal profiles includes the change in the welding traveling speed and heat input.
Figure 1 shows the mapping steps, including thermal mapping on the root and cap pass, leading to HAZ mapping and calculation of yield strength for a broad range of weld traveling speed in the pipe production.
We performed multiple validation tests to compare the prediction with experimental results. We showed that the DT predicts the properties within an acceptable precision, i.e., less than 10 MPa deviation.
Engineering on HAZ Map
Live mapping of HAZ empowers engineers to assess potential weak points in the pipe system after installation. Once the design engineer learns about the service loading condition of the pipeline, commercial computational tools like finite element analysis can precisely predict HAZ behavior under service conditions such as high strain and pipeline landslide applications.
Our algorithm is integrated into the Abaqus computational platform for downstream analysis of pipeline with a built-in HAZ-softening effect, including stress and strain assessment, fracture, fatigue, creep, crash, rupture, vibration, etc.
The algorithm, however, can be tailored to other computational packages. For example, Figure 2 compares a 47-inch (1,194-mm) outer diameter (OD) pipe’s behavior welded with different traveling speeds when the pipe goes under 300 (psi) internal pressure during service.
Slower welding causes more deflection and higher hoop residual stress for transverse crack opening. However, faster welding leads to higher residual stress on the weld cap that facilitates the longitudinal cracking from the outer diameter. Slower welding increases the chance of longitudinal cracking on root pass from the inner diameter.
The API 579 standard allows the fitness-for-service assessment of flaws. This tool builds in the HAZ softening in the evaluation.
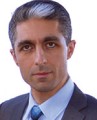
Author: Mahyar Asadi is the manager of advanced welding engineering at Applus+ Canada, focusing on cyber-manufacturing using machine learning, digital twins and simulation tools for welding processes, products and design. He has earned a Ph.D. in computational weld mechanics and is certified as a P.Eng, with expertise IWE designation, ASME FFS, digital twins, and machine learning certificates. He is also an adjunct professor in the Manufacturing Program at the University of British Columbia.
Team members: Sabyasachi Roy, Majid Tanbakuei Kashani and Mathew Smith.
Comments